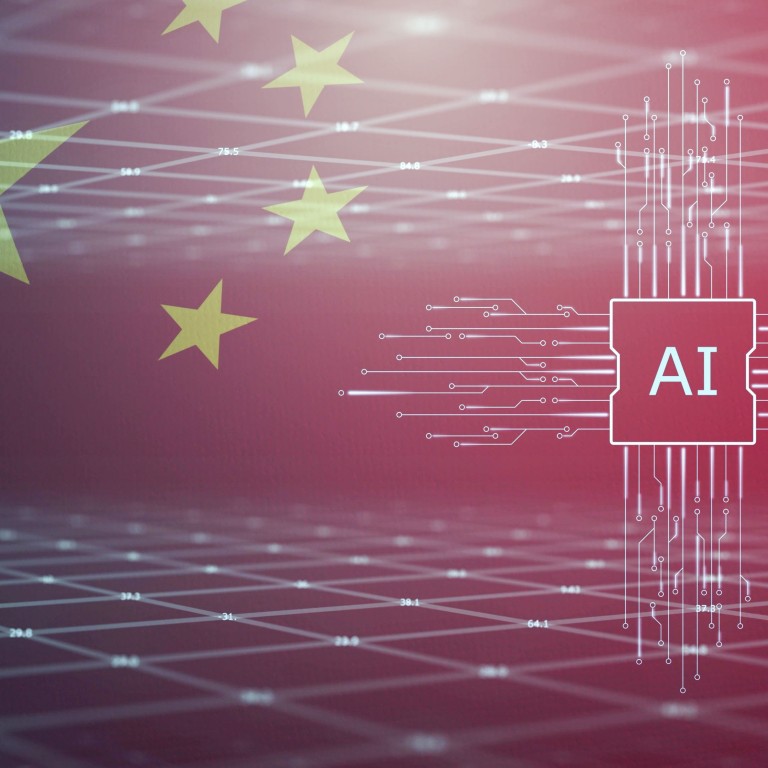
Chinese team creates world’s first AI training system that runs entirely on light
-
-
Taichi-II chip is fully optical and significantly boosts efficiency and performance, according to Tsinghua University scientists
Zhang Tong in Beijing
Published: 6:00am, 9 Aug 2024
A team of scientists in Beijing has developed the world’s first fully optical artificial intelligence chip that they say significantly boosts efficiency and performance.
The Taichi-II chip was created by a Tsinghua University team led by professors Fang Lu and Dai Qionghai, whose study was published in the journal Nature on Wednesday.
It is a major upgrade from their earlier Taichi chip that the researchers in April said had exceeded the energy efficiency of Nvidia’s H100 GPU by over a thousand times.
Training artificial intelligence using the Taichi chip requires the assistance of electronic computers. But Taichi-II can be used for modelling and training based entirely on light – and that has made it much more efficient and improved performance, according to the team.
They said in the paper that the upgrade was a key step for optical computing and could help to take it from the theoretical stage to large-scale experimental applications, as well as address the growing demand for computational power with low energy consumption.
It could also provide an alternative after the US restricted China’s access to the most powerful graphics processing unit, or GPU, chips for AI training.
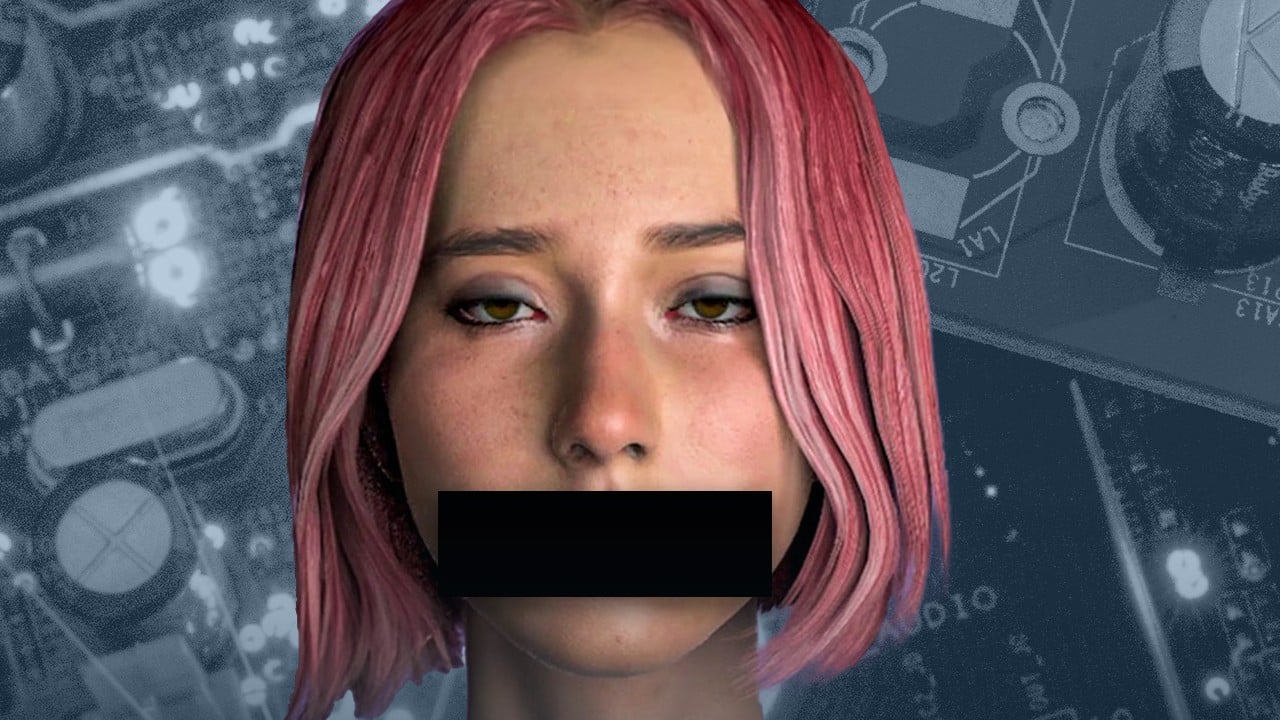
08:54
Is China’s technology falling behind in the race for its own ChatGPT?
Is China’s technology falling behind in the race for its own ChatGPT?
Taichi-II’s performance was found to be superior to its predecessor in various scenarios, according to the paper.
It sped up the training of optical networks with millions of parameters by an order of magnitude and increased the accuracy of classification tasks by 40 per cent.
In the field of complex scenario imaging, Taichi-II’s energy efficiency in low-light environments improved by six orders of magnitude.
Fang said conventional optical AI methods typically involved emulating electronic artificial neural networks on photonic architecture – based on light – designed on electronic computers.
“Because of system imperfections and the complexity of light-wave propagation, perfectly precise modelling of a general optical system is impossible, and a mismatch always occurs between the offline model and the real system,” she said.
To overcome these challenges, the team developed a method where a computer-intensive training process is conducted directly on the optical chip – so most of the machine learning can be carried out in parallel. They called this fully forward mode, or FFM, learning.
“This architecture enables high-precision training and supports large-scale network training,” wrote Xue Zhiwei, lead author of the study and a doctoral student.
FFM learning takes advantage of commercially available high-speed optical modulators and detectors, and it could do better than GPUs in the accelerated learning stakes.
“Our research envisions a future where these chips form the foundation of optical computing power for AI model construction,” Fang said.